您所在的位置:首页 - 热点 - 正文热点
groupby计数
俞文
05-09
【热点】
87人已围观
摘要**Title:UnderstandingthePowerofGroupByinDataAnalysis**Intherealmofdataanalysis,particularlyinthecont
Title: Understanding the Power of GroupBy in Data Analysis
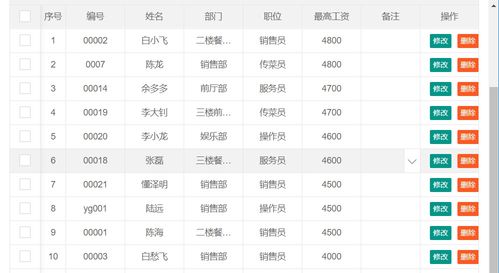
In the realm of data analysis, particularly in the context of programming languages like Python and libraries like pandas, the `groupby` function holds significant importance. This function facilitates the grouping of data based on one or more categorical variables, enabling powerful insights and analysis. Let's delve into the intricacies of `groupby`, its applications, and some best practices for effective utilization.
Introduction to GroupBy:
At its core, the `groupby` operation involves splitting the data into groups based on some criteria, applying a function to each group independently, and then combining the results into a data structure. It's a fundamental operation in data manipulation and aggregation, commonly used in exploratory data analysis, data cleaning, and feature engineering.
Syntax and Basic Usage:
In Python's pandas library, the `groupby` function is typically used in conjunction with aggregation functions such as `sum`, `mean`, `count`, etc. Here's a basic syntax:
```python
grouped = df.groupby('column_name')
result = grouped.agg({'column_name': 'aggregation_function'})
```
Applications of GroupBy:
1.
Descriptive Statistics:
GroupBy allows computing descriptive statistics for subsets of data. For instance, calculating the mean salary by department in a company dataset.2.
Data Exploration:
It aids in exploring relationships between variables. Grouping data by time intervals or categories can reveal trends and patterns.3.
Data Cleaning:
GroupBy facilitates identifying and handling missing values or outliers within specific groups, rather than the entire dataset.4.
Feature Engineering:
Creating new features based on grouped data, such as calculating proportions or ratios within categories.Best Practices:
1.
Choose Appropriate Columns:
Select columns for grouping that provide meaningful insights and align with the analysis objectives.2.
Handle Missing Data:
Decide whether to exclude or impute missing values before performing the GroupBy operation.3.
Consider Performance:
For large datasets, optimize performance by avoiding unnecessary computations and utilizing vectorized operations where possible.4.
Avoid GroupBy on High Cardinality Data:
Grouping on columns with high cardinality may lead to excessive memory usage and slower computations.5.
Explore Multiple Variables:
Experiment with grouping by multiple columns to uncover deeper insights and interactions between variables.Example:
Let's illustrate the usage of GroupBy with a simple example. Consider a dataset of sales transactions:
| Product | Category | Sales |
||||
| A | Electronics | 200 |
| B | Clothing | 150 |
| A | Electronics | 300 |
| C | Electronics | 180 |
| B | Clothing | 250 |
We can group this data by the 'Category' column and calculate the total sales for each category:
```python
grouped = df.groupby('Category')
total_sales = grouped['Sales'].sum()
```
Conclusion:
The `groupby` operation is a powerful tool in a data analyst's arsenal, offering the ability to dissect datasets, derive insights, and make informed decisions. By understanding its mechanics, syntax, and best practices, analysts can leverage GroupBy to its full potential, unlocking deeper understanding and actionable insights from their data.
By mastering GroupBy techniques, analysts can elevate their data analysis capabilities and drive impactful outcomes in various domains, ranging from finance and marketing to healthcare and beyond.
Additional Resources:
[Pandas Documentation](https://pandas.pydata.org/docs/)
[DataCamp GroupBy Tutorial](https://www.datacamp.com/community/tutorials/pandassplitapplycombinegroupby)
[Towards Data Science Article on GroupBy](https://towardsdatascience.com/pandasgroupbyaggregatetransformfilterc95ba3444bbb)
GroupBy opens a gateway to explore and understand data in more profound ways, fostering better decisionmaking and insights across industries and domains.
版权声明: 免责声明:本网站部分内容由用户自行上传,若侵犯了您的权益,请联系我们处理,谢谢!联系QQ:2760375052
上一篇: rank函数的用法是什么
下一篇: dw编程代码大全
最近发表
- 一款值得信赖的全能座驾
- Jeep牧马人,越野传奇的全面解析
- 轻松掌握 XP 中文语言包下载与安装全攻略
- 深入探索Google操作系统,如何改变我们的数字生活
- 一款独特的美式SUV
- 轻松入门电脑知识,畅游数字世界——电脑知识学习网带你全面掌握
- 深入解读vivo Y93手机参数,性能、功能与用户体验
- 电源已接通但未充电?别慌!详解及解决方法
- 苹果SE4上市时间及价格全解析,性价比之王的回归
- 探寻AM3平台的最佳CPU选择
- 别克君威价格全解析,购车必备指南
- 全面解析与深度评测
- 理解负指数分布图像,隐藏在日常生活中的数学之美
- 全面解析与购车指南
- 深入了解标志206最新报价,购车指南与市场分析
- 深入了解 i3 10100,一款适合日常生活的高效处理器
- 走进vivo手机商城,探索智能生活的新篇章
- 5万以下汽车报价大全,为您精选高性价比的经济型车型
- 一辆小车的精彩故事
- 全面解析与购车建议
- 深入了解昊锐1.8T油耗表现及其优化技巧
- 迈腾18T,都市出行的理想伙伴,轻松驾驭每一段旅程
- 桑塔纳新款,传承经典,焕发新生
- 联发科MT6765,智能手机的高效心脏
- 丰田Previa,一款经典MPV的前世今生
- 小学校长受贿近千万,背后的故事与启示
- 探索移动帝国论坛,连接全球移动技术爱好者的桥梁
- 小小的我预售破4000万,一场梦幻童话的奇迹之旅
- 深度解析凯迪拉克CTS(进口),豪华与性能的完美结合
- 揭秘南方人为何更易患鼻咽癌?
- 豪华与性能的完美结合——价格详解及购车指南
- 我是刑警编剧专访,坚持创作初心,不惯市场之风
- 轻松掌握图标文件的奥秘
- 黄圣依在最强大脑中的高知魅力——路透背后的故事
- 微信紧急提醒,警惕木马病毒——如何防范与应对网络攻击?
- Jeep新大切诺基,经典与现代的完美融合
- 顾客用餐时打火机不慎落入锅内引发爆炸事件解析
- 解读大捷龙报价,购车前必知的关键信息
- 大学生作业中的AI气息,新时代的学习变革
- 比亚迪思锐,探索未来汽车科技的先锋
- 警惕串联他人越级走访,数人多次煽动行为终被抓获的警示
- 经典与现代的完美融合——联想ThinkPad X201,一款改变工作方式的笔记本电脑
- 北京平谷再现鸟中老虎
- 一位七旬官员的人生转折,公诉背后的故事与深思
- 财神鱼离奇死亡,男子悲痛之余做出惊人决定,起锅烧油含泪吃下
- 掌握 Flash 课件制作,从零开始的实用教程
- 蜜雪冰城的新动作,背后的战略调整与市场应对
- 警惕网络谣言,重庆小女孩急需救助的真相揭秘
- 深入了解2012款锋范,经典小车的完美演绎
- 刘诗诗,淡然面对传闻,专注自我成长