您所在的位置:首页 - 热点 - 正文热点
c#围棋
淼幼
04-22
【热点】
524人已围观
摘要**Title:ExploringCUDAProgrammingforGo(围棋)****IntroductiontoCUDAProgrammingforGo(围棋)**CUDA(ComputeUni
Title: Exploring CUDA Programming for Go (围棋)
Introduction to CUDA Programming for Go (围棋)
CUDA (Compute Unified Device Architecture) is a parallel computing platform and application programming interface (API) model created by NVIDIA. It allows developers to utilize the power of NVIDIA GPUs for generalpurpose processing tasks, including accelerating computeintensive applications like artificial intelligence, scientific simulations, and gaming.
In the context of Go (围棋), a game known for its complexity and extensive search space, CUDA programming can offer significant performance improvements, particularly in tasks such as game tree search, pattern recognition, and neural network evaluation. In this guide, we'll explore the fundamentals of CUDA programming for Go (围棋) and how it can be leveraged to enhance gameplaying algorithms.
Understanding CUDA Programming Basics
CUDA programming involves writing code that executes on NVIDIA GPUs. It utilizes a combination of C/C language extensions and runtime libraries to manage the execution of parallel tasks on the GPU. Here's a brief overview of key concepts in CUDA programming:
1.
Kernel Functions
: These are functions that are executed in parallel on the GPU. They are written using CUDA C/C syntax and are launched from the CPU.2.
Thread Hierarchy
: CUDA organizes threads into a hierarchy consisting of grids, blocks, and threads. This hierarchy allows for efficient management of parallel execution.3.
Memory Management
: CUDA provides specialized memory spaces, including global memory, shared memory, and constant memory, which can be accessed by kernel functions.4.
CUDA Libraries
: NVIDIA provides libraries optimized for various tasks, such as cuBLAS for linear algebra operations and cuDNN for deep neural networks.Applying CUDA to Go (围棋) Programming
Now, let's discuss how CUDA programming can be applied to enhance Go (围棋) playing algorithms:
1.
Parallel Search Algorithms
: One of the most computationally intensive tasks in Go (围棋) playing engines is the search algorithm, which explores possible moves and evaluates resulting board positions. By implementing the search algorithm using CUDA kernel functions, it's possible to execute multiple search branches simultaneously on the GPU, leading to significant speedups.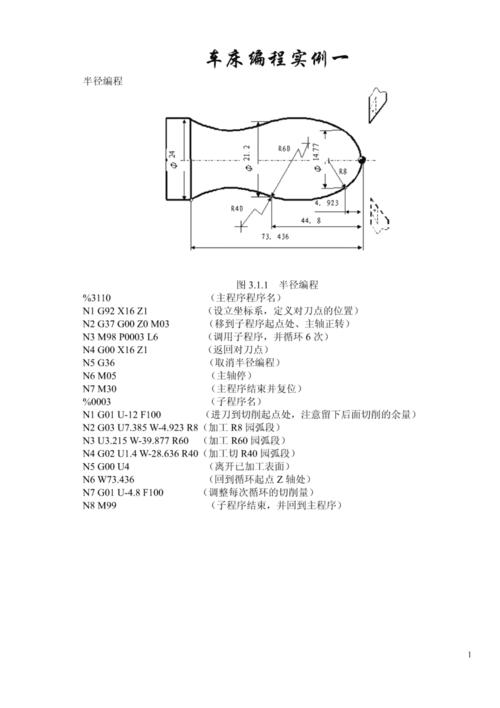
2.
Pattern Recognition
: Go (围棋) relies heavily on pattern recognition, identifying recurring shapes and configurations on the board. CUDA can accelerate pattern matching algorithms by parallelizing the search process across multiple threads, allowing for faster identification of key patterns.3.
Neural Network Evaluation
: Many modern Go (围棋) playing engines use neural networks to evaluate board positions and make move predictions. CUDA enables efficient execution of neural network inference tasks on the GPU, leveraging its parallel processing capabilities to evaluate multiple board positions simultaneously.4.
Monte Carlo Tree Search (MCTS)
: MCTS is a popular algorithm used in Go (围棋) playing engines for decision making. CUDA can accelerate MCTS simulations by parallelizing the rollout phase, where multiple simulations are run in parallel to estimate the value of different moves.Best Practices for CUDA Programming in Go (围棋)
When integrating CUDA into Go (围棋) playing engines, consider the following best practices:
1.
Optimize Memory Access
: Minimize data transfers between the CPU and GPU by optimizing memory access patterns and utilizing shared memory where possible.2.
Thread Divergence
: Avoid thread divergence within CUDA kernels to maximize parallelism and GPU utilization.3.
Profile and Benchmark
: Use profiling tools provided by NVIDIA, such as NVIDIA Visual Profiler, to identify performance bottlenecks and optimize CUDA code accordingly.4.
Error Handling
: Properly handle errors and exceptions in CUDA code to ensure robustness and stability of the application.Conclusion
CUDA programming offers exciting opportunities for enhancing Go (围棋) playing algorithms by leveraging the parallel processing power of NVIDIA GPUs. By implementing parallel search algorithms, accelerating pattern recognition, optimizing neural network evaluation, and speeding up Monte Carlo Tree Search simulations, CUDA can significantly improve the performance of Go (围棋) playing engines. However, it's essential to follow best practices and optimize CUDA code for efficient execution on the GPU. With careful integration and optimization, CUDA can unlock new levels of performance and sophistication in Go (围棋) playing AI systems.
Tags: 类似斯巴达克斯的美剧 激战2论坛 折磨小美美 洛克王国图瑞斯 孔明借东风
版权声明: 免责声明:本网站部分内容由用户自行上传,若侵犯了您的权益,请联系我们处理,谢谢!联系QQ:2760375052
最近发表
- Jeep牧马人,越野传奇的全面解析
- 轻松掌握 XP 中文语言包下载与安装全攻略
- 深入探索Google操作系统,如何改变我们的数字生活
- 一款独特的美式SUV
- 轻松入门电脑知识,畅游数字世界——电脑知识学习网带你全面掌握
- 深入解读vivo Y93手机参数,性能、功能与用户体验
- 电源已接通但未充电?别慌!详解及解决方法
- 苹果SE4上市时间及价格全解析,性价比之王的回归
- 探寻AM3平台的最佳CPU选择
- 别克君威价格全解析,购车必备指南
- 全面解析与深度评测
- 理解负指数分布图像,隐藏在日常生活中的数学之美
- 全面解析与购车指南
- 深入了解标志206最新报价,购车指南与市场分析
- 深入了解 i3 10100,一款适合日常生活的高效处理器
- 走进vivo手机商城,探索智能生活的新篇章
- 5万以下汽车报价大全,为您精选高性价比的经济型车型
- 一辆小车的精彩故事
- 全面解析与购车建议
- 深入了解昊锐1.8T油耗表现及其优化技巧
- 迈腾18T,都市出行的理想伙伴,轻松驾驭每一段旅程
- 桑塔纳新款,传承经典,焕发新生
- 联发科MT6765,智能手机的高效心脏
- 丰田Previa,一款经典MPV的前世今生
- 小学校长受贿近千万,背后的故事与启示
- 探索移动帝国论坛,连接全球移动技术爱好者的桥梁
- 小小的我预售破4000万,一场梦幻童话的奇迹之旅
- 深度解析凯迪拉克CTS(进口),豪华与性能的完美结合
- 揭秘南方人为何更易患鼻咽癌?
- 豪华与性能的完美结合——价格详解及购车指南
- 我是刑警编剧专访,坚持创作初心,不惯市场之风
- 轻松掌握图标文件的奥秘
- 黄圣依在最强大脑中的高知魅力——路透背后的故事
- 微信紧急提醒,警惕木马病毒——如何防范与应对网络攻击?
- Jeep新大切诺基,经典与现代的完美融合
- 顾客用餐时打火机不慎落入锅内引发爆炸事件解析
- 解读大捷龙报价,购车前必知的关键信息
- 大学生作业中的AI气息,新时代的学习变革
- 比亚迪思锐,探索未来汽车科技的先锋
- 警惕串联他人越级走访,数人多次煽动行为终被抓获的警示
- 经典与现代的完美融合——联想ThinkPad X201,一款改变工作方式的笔记本电脑
- 北京平谷再现鸟中老虎
- 一位七旬官员的人生转折,公诉背后的故事与深思
- 财神鱼离奇死亡,男子悲痛之余做出惊人决定,起锅烧油含泪吃下
- 掌握 Flash 课件制作,从零开始的实用教程
- 蜜雪冰城的新动作,背后的战略调整与市场应对
- 警惕网络谣言,重庆小女孩急需救助的真相揭秘
- 深入了解2012款锋范,经典小车的完美演绎
- 刘诗诗,淡然面对传闻,专注自我成长
- 开启搜索引擎优化与数字营销的新旅程